
Metabolome

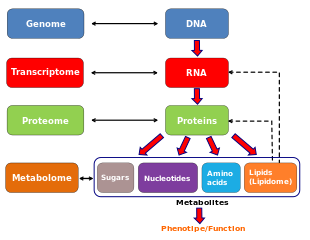
The metabolome refers to the complete set of small-molecule chemicals found within a biological sample.[1] The biological sample can be a cell, a cellular organelle, an organ, a tissue, a tissue extract, a biofluid or an entire organism. The small molecule chemicals found in a given metabolome may include both endogenous metabolites that are naturally produced by an organism (such as amino acids, organic acids, nucleic acids, fatty acids, amines, sugars, vitamins, co-factors, pigments, antibiotics, etc.) as well as exogenous chemicals (such as drugs, environmental contaminants, food additives, toxins and other xenobiotics) that are not naturally produced by an organism.[2][3]

In other words, there is both an endogenous metabolome and an exogenous metabolome. The endogenous metabolome can be further subdivided to include a "primary" and a "secondary" metabolome (particularly when referring to plant or microbial metabolomes). A primary metabolite is directly involved in the normal growth, development, and reproduction. A secondary metabolite is not directly involved in those processes, but usually has important ecological function. Secondary metabolites may include pigments, antibiotics or waste products derived from partially metabolized xenobiotics. The study of the metabolome is called metabolomics.

Origins
The word metabolome appears to be a blending of the words "metabolite" and "chromosome". It was constructed to imply that metabolites are indirectly encoded by genes or act on genes and gene products. The term "metabolome" was first used in 1998 [1][4] and was likely coined to match with existing biological terms referring to the complete set of genes (the genome), the complete set of proteins (the proteome) and the complete set of transcripts (the transcriptome). The first book on metabolomics was published in 2003.[5] The first journal dedicated to metabolomics (titled simply "Metabolomics") was launched in 2005 and is currently edited by Prof. Roy Goodacre. Some of the more significant early papers on metabolome analysis are listed in the references below.[6][7][8][9]

Measuring the metabolome
The metabolome reflects the interaction between an organism's genome and its environment. As a result, an organism's metabolome can serve as an excellent probe of its phenotype (i.e. the product of its genotype and its environment). Metabolites can be measured (identified, quantified or classified) using a number of different technologies including NMR spectroscopy and mass spectrometry.[10] Most mass spectrometry (MS) methods must be coupled to various forms of liquid chromatography (LC), gas chromatography (GC) or capillary electrophoresis (CE) to facilitate compound separation. Each method is typically able to identify or characterize 50-5,000 different metabolites or metabolite "features" at a time, depending on the instrument or protocol being used. Currently it is not possible to analyze the entire range of metabolites by a single analytical method.

Nuclear magnetic resonance (NMR) spectroscopy is an analytical chemistry technique that measures the absorption of radiofrequency radiation of specific nuclei when molecules containing those nuclei are placed in strong magnetic fields. The frequency (i.e. the chemical shift) at which a given atom or nucleus absorbs is highly dependent on the chemical environment (bonding, chemical structure nearest neighbours, solvent) of that atom in a given molecule. The NMR absorption patterns produce "resonance" peaks at different frequencies or different chemical shifts – this collection of peaks is called an NMR spectrum. Because each chemical compound has a different chemical structure, each compound will have a unique (or almost unique) NMR spectrum. As a result, NMR is particularly useful for the characterization, identification and quantification of small molecules, such as metabolites. The widespread use of NMR for "classical" metabolic studies, along with its exceptional capacity to handle complex metabolite mixtures is likely the reason why NMR was one of the first technologies to be widely adopted for routine metabolome measurements. As an analytical technique, NMR is non-destructive, non-biased, easily quantifiable, requires little or no separation, permits the identification of novel compounds and it needs no chemical derivatization. NMR is particularly amenable to detecting compounds that are less tractable to LC-MS analysis, such as sugars, amines or volatile liquids or GC-MS analysis, such as large molecules (>500 Da) or relatively non-reactive compounds. NMR is not a very sensitive technique with a lower limit of detection of about 5 μM. Typically 50-150 compounds can be identified by NMR-based metabolomic studies.

Mass spectrometry is an analytical technique that measures the mass-to-charge ratio of molecules. Molecules or molecular fragments are typically charged or ionized by spraying them through a charged field (electrospray ionization), bombarding them with electrons from a hot filament (electron ionization) or blasting them with a laser when they are placed on specially coated plates (matrix assisted laser desorption ionization). The charged molecules are then propelled through space using electrodes or magnets and their speed, rate of curvature, or other physical characteristics are measured to determine their mass-to-charge ratio. From these data the mass of the parent molecule can be determined. Further fragmentation of the molecule through controlled collisions with gas molecules or with electrons can help determine the structure of molecules. Very accurate mass measurements can also be used to determine the elemental formulas or elemental composition of compounds. Most forms of mass spectrometry require some form of separation using liquid chromatography or gas chromatography. This separation step is required to simplify the resulting mass spectra and to permit more accurate compound identification. Some mass spectrometry methods also require that the molecules be derivatized or chemically modified so that they are more amenable for chromatographic separation (this is particularly true for GC-MS). As an analytical technique, MS is a very sensitive method that requires very little sample (<1 ng of material or <10 μL of a biofluid) and can generate signals for thousands of metabolites from a single sample. MS instruments can also be configured for very high throughput metabolome analyses (hundreds to thousands of samples a day). Quantification of metabolites and the characterization of novel compound structures is more difficult by MS than by NMR. LC-MS is particularly amenable to detecting hydrophobic molecules (lipids, fatty acids) and peptides while GC-MS is best for detecting small molecules (<500 Da) and highly volatile compounds (esters, amines, ketones, alkanes, thiols).

Unlike the genome or even the proteome, the metabolome is a highly dynamic entity that can change dramatically, over a period of just seconds or minutes. As a result, there is growing interest in measuring metabolites over multiple time periods or over short time intervals using modified versions of NMR or MS-based metabolomics.

Metabolome databases
Because an organism's metabolome is largely defined by its genome, different species will have different metabolomes. Indeed, the fact that the metabolome of a tomato is different from the metabolome of an apple is the reason why these two fruits taste so different. Furthermore, different tissues, different organs and biofluids associated with those organs and tissues can also have distinctly different metabolomes. The fact that different organisms and different tissues/biofluids have such different metabolomes has led to the development of a number of organism-specific and biofluid-specific metabolome databases. Some of the better known metabolome databases include the Human Metabolome Database or HMDB,[11] the Yeast Metabolome Database or YMDB,[12] the E. coli Metabolome Database or ECMDB,[13] the Arabidopsis metabolome database or AraCyc [14] as well as the Urine Metabolome Database,[15] the Cerebrospinal Fluid (CSF) Metabolome Database [16] and the Serum Metabolome Database.[17] The latter three databases are specific to human biofluids. A number of very popular general metabolite databases also exist including KEGG,[18] MetaboLights,[19] the Golm Metabolome Database,[20] MetaCyc,[21] LipidMaps[22] and Metlin.[23] Metabolome databases can be distinguished from metabolite databases in that metabolite databases contain lightly annotated or synoptic metabolite data from multiple organisms while metabolome databases contain richly detailed and heavily referenced chemical, pathway, spectral and metabolite concentration data for specific organisms.
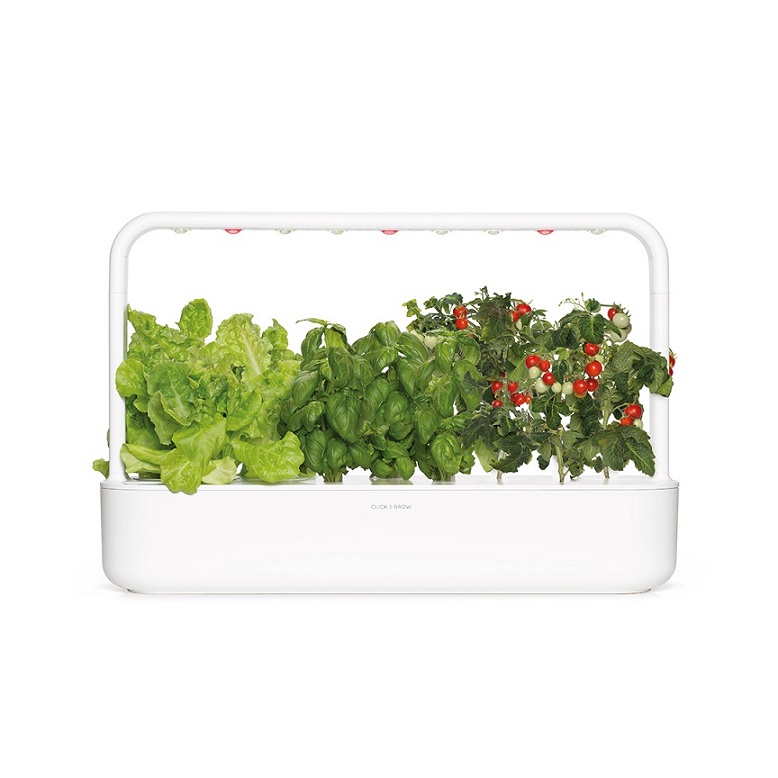
The Human Metabolome Database
The Human Metabolome Database (HMDB) is a freely available, open-access database containing detailed data on more than 40,000 metabolites that have already been identified or are likely to be found in the human body. The HMDB contains three kinds of information:

- Chemical information,
- Clinical information and
- Biochemical information.
The chemical data includes >40,000 metabolite structures with detailed descriptions, extensive chemical classifications, synthesis information and observed/calculated chemical properties. It also contains nearly 10,000 experimentally measured NMR, GC-MS and LC/MS spectra from more than 1,100 different metabolites. The clinical information includes data on >10,000 metabolite-biofluid concentrations, metabolite concentration information on more than 600 different human diseases and pathway data for more than 200 different inborn errors of metabolism. The biochemical information includes nearly 6,000 protein (and DNA) sequences and more than 5,000 biochemical reactions that are linked to these metabolite entries. The HMDB supports a wide variety of online queries including text searches, chemical structure searches, sequence similarity searches and spectral similarity searches. This makes it particularly useful for metabolomic researchers who are attempting to identify or understand metabolites in clinical metabolomic studies. The first version of the HMDB was released in Jan. 1 2007 and was compiled by scientists at the University of Alberta and the University of Calgary. At that time, they reported data on 2,500 metabolites, 1,200 drugs and 3,500 food components. Since then these scientists have greatly expanded the collection. The version 3.5 of the HMDB contains >16,000 endogenous metabolites, >1,500 drugs and >22,000 food constituents or food metabolites.[24]

Human biofluid metabolomes
Scientists at the University of Alberta have been systematically characterizing specific biofluid metabolomes including the serum metabolome,[17] the urine metabolome,[15] the cerebrospinal fluid (CSF) metabolome [16] and the saliva metabolome. These efforts have involved both experimental metabolomic analysis (involving NMR, GC-MS, ICP-MS, LC-MS and HPLC assays) as well as extensive literature mining. According to their data, the human serum metabolome contains at least 4,200 different compounds (including many lipids), the human urine metabolome contains at least 3,000 different compounds (including hundreds of volatiles and gut microbial metabolites), the human CSF metabolome contains nearly 500 different compounds while the human saliva metabolome contains approximately 400 different metabolites, including many bacterial products.

Yeast Metabolome Database
The Yeast Metabolome Database is a freely accessible, online database of >2,000 small molecule metabolites found in or produced by Saccharomyces cerevisiae (Baker's yeast). The YMDB contains two kinds of information:

- Chemical information and
- Biochemical information.
The chemical information in YMDB includes 2,027 metabolite structures with detailed metabolite descriptions, extensive chemical classifications, synthesis information and observed/calculated chemical properties. It also contains nearly 4,000 NMR, GC-MS and LC/MS spectra obtained from more than 500 different metabolites. The biochemical information in YMDB includes >1,100 protein (and DNA) sequences and >900 biochemical reactions. The YMDB supports a wide variety of queries including text searches, chemical structure searches, sequence similarity searches and spectral similarity searches. This makes it particularly useful for metabolomic researchers who are studying yeast as a model organism or who are looking into optimizing the production of fermented beverages (wine, beer).

Secondary electrospray ionization-high resolution mass spectrometry SESI-HRMS is a non-invasive analytical technique that allows us to monitor the yeast metabolic activities. SESI-HRMS has found around 300 metabolites in the yeast fermentation process, this suggests that a large number of glucose metabolites are not reported in the literature.[25]

The Escherichia coli Metabolome Database
The E. Coli Metabolome Database is a freely accessible, online database of >2,700 small molecule metabolites found in or produced by Escherichia coli (E. coli strain K12, MG1655). The ECMDB contains two kinds of information:

- Chemical information and
- Biochemical information.
The chemical information includes more than 2,700 metabolite structures with detailed metabolite descriptions, extensive chemical classifications, synthesis information and observed/calculated chemical properties. It also contains nearly 5,000 NMR, GC-MS and LC-MS spectra from more than 600 different metabolites. The biochemical information includes >1,600 protein (and DNA) sequences and >3,100 biochemical reactions that are linked to these metabolite entries. The ECMDB supports many different types of online queries including text searches, chemical structure searches, sequence similarity searches and spectral similarity searches. This makes it particularly useful for metabolomic researchers who are studying E. coli as a model organism.

Secondary electrospray ionization (SESI-MS) can discriminate between eleven E. Coli strains thanks to the volatile organic compound profiling.[26]

Metabolome atlas of the aging mouse brain
In 2021, the first brain metabolome atlas of the mouse brain – and of an animal (a mammal) across different life stages – was released online. The data differentiates by brain regions and the metabolic changes could be "mapped to existing gene and protein brain atlases".[27][28]

Intestinal metabolome
Human intestinal microbiota contribute to the etiology of colorectal cancer via their metabolome.[29] In particular, the conversion of primary bile acids to secondary bile acids as a consequence of bacterial metabolism in the colon promotes carcinogenesis.[29]

See also
References
- ^ a b Oliver SG, Winson MK, Kell DB, Baganz F (September 1998). "Systematic functional analysis of the yeast genome". Trends in Biotechnology. 16 (9): 373–8. CiteSeerX 10.1.1.33.5221. doi:10.1016/S0167-7799(98)01214-1. PMID 9744112.
- ^ Wishart DS (September 2007). "Current progress in computational metabolomics". Briefings in Bioinformatics. 8 (5): 279–93. doi:10.1093/bib/bbm030. PMID 17626065.
- ^ Nordström A, O'Maille G, Qin C, Siuzdak G (May 2006). "Nonlinear data alignment for UPLC-MS and HPLC-MS based metabolomics: quantitative analysis of endogenous and exogenous metabolites in human serum". Analytical Chemistry. 78 (10): 3289–95. doi:10.1021/ac060245f. PMC 3705959. PMID 16689529.
- ^ Tweeddale H, Notley-McRobb L, Ferenci T (October 1998). "Effect of slow growth on metabolism of Escherichia coli, as revealed by global metabolite pool ("metabolome") analysis". Journal of Bacteriology. 180 (19): 5109–16. doi:10.1128/JB.180.19.5109-5116.1998. PMC 107546. PMID 9748443.
- ^ Harrigan GG, Goodacre R, eds. (2003). Metabolic Profiling: Its Role in Biomarker Discovery and Gene Function Analysis. Boston: Kluwer Academic Publishers. ISBN 978-1-4020-7370-0.
- ^ Fiehn O, Kloska S, Altmann T (February 2001). "Integrated studies on plant biology using multiparallel techniques". Current Opinion in Biotechnology. 12 (1): 82–6. doi:10.1016/S0958-1669(00)00165-8. PMID 11167078.
- ^ Fiehn O (2001). "Combining genomics, metabolome analysis, and biochemical modelling to understand metabolic networks". Comparative and Functional Genomics. 2 (3): 155–68. doi:10.1002/cfg.82. PMC 2447208. PMID 18628911.
- ^ Weckwerth W (2003). "Metabolomics in systems biology". Annual Review of Plant Biology. 54: 669–89. doi:10.1146/annurev.arplant.54.031902.135014. PMID 14503007. S2CID 1197884.
- ^ Goodacre R, Vaidyanathan S, Dunn WB, Harrigan GG, Kell DB (May 2004). "Metabolomics by numbers: acquiring and understanding global metabolite data". Trends in Biotechnology. 22 (5): 245–52. doi:10.1016/j.tibtech.2004.03.007. PMID 15109811.
- ^ Lu W, Su X, Klein MS, Lewis IA, Fiehn O, Rabinowitz JD (June 2017). "Metabolite Measurement: Pitfalls to Avoid and Practices to Follow". Annual Review of Biochemistry. 86 (1): 277–304. doi:10.1146/annurev-biochem-061516-044952. PMC 5734093. PMID 28654323.
- ^ Wishart DS, Tzur D, Knox C, Eisner R, Guo AC, Young N, et al. (January 2007). "HMDB: the Human Metabolome Database". Nucleic Acids Research. 35 (Database issue): D521-6. doi:10.1093/nar/gkl923. PMC 1899095. PMID 17202168.
- ^ Jewison T, Knox C, Neveu V, Djoumbou Y, Guo AC, Lee J, et al. (January 2012). "YMDB: the Yeast Metabolome Database". Nucleic Acids Research. 40 (Database issue): D815-20. doi:10.1093/nar/gkr916. PMC 3245085. PMID 22064855.
- ^ Guo AC, Jewison T, Wilson M, Liu Y, Knox C, Djoumbou Y, et al. (January 2013). "ECMDB: the E. coli Metabolome Database". Nucleic Acids Research. 41 (Database issue): D625-30. doi:10.1093/nar/gks992. PMC 3531117. PMID 23109553.
- ^ Mueller LA, Zhang P, Rhee SY (June 2003). "AraCyc: a biochemical pathway database for Arabidopsis". Plant Physiology. 132 (2): 453–60. doi:10.1104/pp.102.017236. PMC 166988. PMID 12805578.
- ^ a b Bouatra S, Aziat F, Mandal R, Guo AC, Wilson MR, Knox C, et al. (Sep 2013). "The human urine metabolome". PLOS ONE. 8 (9): e73076. Bibcode:2013PLoSO...873076B. doi:10.1371/journal.pone.0073076. PMC 3762851. PMID 24023812.
- ^ a b Mandal R, Guo AC, Chaudhary KK, Liu P, Yallou FS, Dong E, et al. (April 2012). "Multi-platform characterization of the human cerebrospinal fluid metabolome: a comprehensive and quantitative update". Genome Medicine. 4 (4): 38. doi:10.1186/gm337. PMC 3446266. PMID 22546835.
- ^ a b Psychogios N, Hau DD, Peng J, Guo AC, Mandal R, Bouatra S, et al. (February 2011). "The human serum metabolome". PLOS ONE. 6 (2): e16957. Bibcode:2011PLoSO...616957P. doi:10.1371/journal.pone.0016957. PMC 3040193. PMID 21359215.
- ^ Kanehisa M, Goto S (January 2000). "KEGG: kyoto encyclopedia of genes and genomes". Nucleic Acids Research. 28 (1): 27–30. doi:10.1093/nar/28.1.27. PMC 102409. PMID 10592173.
- ^ Haug K, Salek RM, Conesa P, Hastings J, de Matos P, Rijnbeek M, et al. (January 2013). "MetaboLights--an open-access general-purpose repository for metabolomics studies and associated meta-data". Nucleic Acids Research. 41 (Database issue): D781-6. doi:10.1093/nar/gks1004. PMC 3531110. PMID 23109552.
- ^ Kopka J, Schauer N, Krueger S, Birkemeyer C, Usadel B, Bergmüller E, et al. (April 2005). "[email protected]: the Golm Metabolome Database". Bioinformatics. 21 (8): 1635–8. doi:10.1093/bioinformatics/bti236. hdl:20.500.11850/33179. PMID 15613389.
- ^ Caspi R, Altman T, Dale JM, Dreher K, Fulcher CA, Gilham F, et al. (January 2010). "The MetaCyc database of metabolic pathways and enzymes and the BioCyc collection of pathway/genome databases". Nucleic Acids Research. 38 (Database issue): D473-9. doi:10.1093/nar/gkp875. PMC 2808959. PMID 19850718.
- ^ Fahy E, Sud M, Cotter D, Subramaniam S (July 2007). "LIPID MAPS online tools for lipid research". Nucleic Acids Research. 35 (Web Server issue): W606-12. doi:10.1093/nar/gkm324. PMC 1933166. PMID 17584797.
- ^ Smith CA, O'Maille G, Want EJ, Qin C, Trauger SA, Brandon TR, et al. (December 2005). "METLIN: a metabolite mass spectral database". Therapeutic Drug Monitoring. 27 (6): 747–51. doi:10.1097/01.ftd.0000179845.53213.39. PMID 16404815. S2CID 14774455.
- ^ Wishart DS, Jewison T, Guo AC, Wilson M, Knox C, Liu Y, et al. (January 2013). "HMDB 3.0--The Human Metabolome Database in 2013". Nucleic Acids Research. 41 (Database issue): D801-7. doi:10.1093/nar/gks1065. PMC 3531200. PMID 23161693.
- ^ Tejero Rioseras A, Garcia Gomez D, Ebert BE, Blank LM, Ibáñez AJ, Sinues PM (October 2017). "Comprehensive Real-Time Analysis of the Yeast Volatilome". Scientific Reports. 7 (1): 14236. Bibcode:2017NatSR...714236T. doi:10.1038/s41598-017-14554-y. PMC 5660155. PMID 29079837.
- ^ Zhu J, Hill JE (June 2013). "Detection of Escherichia coli via VOC profiling using secondary electrospray ionization-mass spectrometry (SESI-MS)". Food Microbiology. 34 (2): 412–7. doi:10.1016/j.fm.2012.12.008. PMC 4425455. PMID 23541210.
- ^ "A map of mouse brain metabolism in aging". UC Davis. Retrieved 15 November 2021.
- ^ Ding J, Ji J, Rabow Z, Shen T, Folz J, Brydges CR, et al. (October 2021). "A metabolome atlas of the aging mouse brain". Nature Communications. 12 (1): 6021. Bibcode:2021NatCo..12.6021D. doi:10.1038/s41467-021-26310-y. PMC 8519999. PMID 34654818.
- ^ a b Louis P, Hold GL, Flint HJ (October 2014). "The gut microbiota, bacterial metabolites and colorectal cancer". Nature Reviews. Microbiology. 12 (10): 661–72. doi:10.1038/nrmicro3344. PMID 25198138.
External links
See what we do next...
OR
By submitting your email or phone number, you're giving mschf permission to send you email and/or recurring marketing texts. Data rates may apply. Text stop to cancel, help for help.
Success: You're subscribed now !